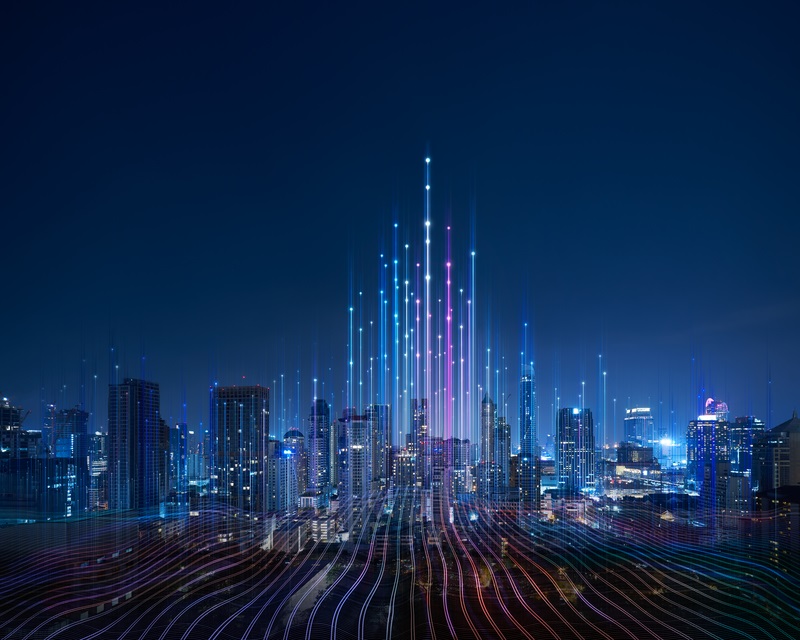
Photo: Jamesteohart | Dreamstime.com
Introducing the Neural Smart City Model
10 January 2022
By Renato de Castro and Ross McKenzie, Executive Directors at RMA Advisory
We have been hearing about ‘smart cities’ since the late 1960s. In the last decade various attempts have been made to better define the term, and in the last five years in particular, cities worldwide have been assessed, listed and awarded in a range of rankings that tried to show their technological advances and, consequently, to quantify improvement in the quality of life of their people. This was all theoretically interesting, and often romanticised, even utopian, but there was always doubt, confusion and uncertainty about what ‘smart’ really meant.
When 2020 and the pandemic arrived, it became clear that cities were not as smart or resilient as we wanted to believe. This trigger resurfaced some of the most recurrent questions among scholars of the smart city concept, and reignited the most sceptical critics: “Smart Cities are dead”; “It was just a fad”; “It was all just marketing”; “The Emperor has no clothes!” and so on.
Applying straightforward, cause-effect analyses, one could conclude they are not entirely wrong. Against expectations, smart cities have failed in many ways, we agree, but the underlying reasons and reality are not so straightforward. The concept was not, and is not, flawed or purely marketing. The reality is that it has been misunderstood, over-simplified and looked at too short-sightedly.
This is normal and natural. In the long history of scientific and societal achievement, the development of complex concepts evolves as knowledge and understanding grows.
Multifaceted models
To date, the models have been enough to support the basic control and organisation of city administration to solve, or at least mitigate, urban challenges. This was not all wrong or wasted, but cities have evolved, and so must the concept. Many people still believe that a smart city is simply synonymous with technology.
Matrix models, a general theory from 2000 onwards and entirely well accepted before the Covid-19 pandemic, have been relied upon, which made sense to a point. In practical terms, matrix structures are perfect for illustrating the relationships between two or more variables and, especially, creating models or patterns that can be reapplied. Thus, for more than two decades benchmarking against these models has been the primary practical tool in implementing the “smart city kit”.
The causal model and the thesis of representing smart cities as matrix structures were thus generally correct, but they did not answer all the questions, and the IoT revolution has further stretched their suitability to represent the complexity of the modern smart city ecosystem. They were part of the organic evolution, our cities’ journey. As a result, we built cities that were claiming to be smart, but have been perceived as failures. In the first major crisis of their era, whilst some had some limited successes (for example using city-wide data sets), none have lived up to the promises or expectations. Real resilience was missing, not because of a lack of planning or implementing initiatives, but mainly because of limitations in properly understanding and modelling the system. Our cities, however ‘smart’, are dynamic ecosystems; we believe they are sophisticated ‘neural’ networks.
The Neural concept
The 2016 City SmartUp concept already advocated a new perspective in modelling smarter cities. The proposal was to use the start-up management model to develop smart city policies and projects. The methodology, successfully applied in cities worldwide and, complemented by the Smart City Wheel model and the ISO 37.100 series, serves as the basis for the new concept we present now, developed over the last 18 months: the Neural Smart City Model.
To explain the thesis, we start with the etymology of the term smart city. The word smart is generally related to ‘intelligence’. The Oxford English Dictionary defines intelligence as the ability to understand and resolve new problems and conflicts and to adapt to new situations. If human intelligence is thus summarised as reasoning, problem-solving, and learning, it can be seen that using the term smart city to describe urban evolution makes sense. We were on the right path but probably with the wrong map.
The Neural Model proposes that the interrelationship between the various pillars of a city, such as health, education, security, and sanitation, among others, and the technologies used to solve urban problems, is analogous to the complex connections in neural networks. As in the human brain, urban intelligence derives from the combination of, and connections between, reasoning and logical analysis, the orientation of solutions, and our cities’ capacity to learn from experiences. Neural connections are also not necessarily made in a constant sequential, standardised, or repetitive manner. On the contrary, once any fundamental element in the interrelation of variables is altered, the neural connections are remade, seeking a new path, ratifying the reasoning-resolution-learning model. That is how we evolved as a species, and we believe that is how our cities become smarter in reality.
Mapping the city
In practical terms, The Neural Model proposes six essential layers for helping to understand and develop smart city policies and solutions:
- City DNA
- The Smart City Dimensions
- The Foundational (or base, core, essential) Technologies
- The Contemporary Urban Pillars
- The Edge Technologies
- Use cases
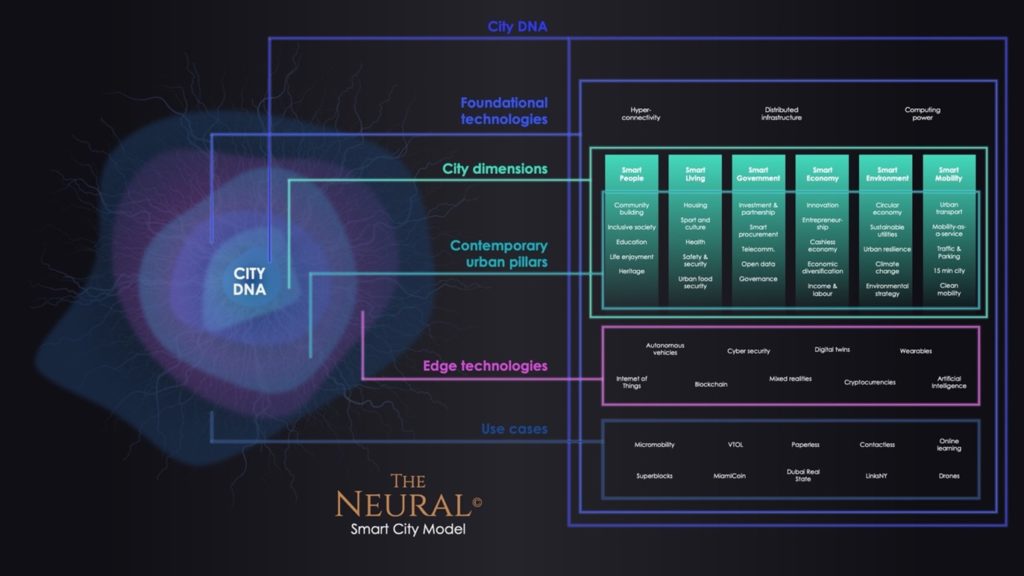
There are two important factors in understanding, and ultimately applying, the Neural Model:
- One must always work from the centre (the unique DNA of each city), to the extremities – indeed one of the biggest limitations with previous models was the tendency to allow a ‘solution first’ approach, before understanding properly the true character of the underlying city.
- The further the layer from the centre, the more volatile it is. As with human DNA, the centre is fixed, immutable as the essence of the city, while cutting-edge technologies and use cases at the outermost layers vary according to context, and evolve and expand, reacting quickly to external and environmental changes and technological development.
With this new model, we begin to understand why many smart city projects developed under previous frameworks have failed. Without considering the local ecosystem’s unique aspects; systematically building layers based on contextual analysis; prioritising and investing in the truly foundational technologies of connectivity, distributed infrastructure and computing power; and acknowledging that edge and use-case technologies are a dynamic, evolving system, projects where stakeholders focus on solving tactical problems by trying to benchmark or copy from other cities are doomed to failure. They are not relevant, robust or future-proofed. For example, are we putting more effort into implementing an artificial intelligence app or a pilot project of autonomous vehicles, instead of guaranteeing high quality internet for everyone?
Are smart cities worldwide a failure? A fad? We believe not. The problem was that we had not appreciated, or been willing to acknowledge, the multifarious nature of the topic, and so had not found the right model to capture the complexity. By considering the layers and interactions that have always existed in smart cities as akin to neural connections, we believe that city stakeholders will better be able to manage this complexity, thus better serving the diverse needs of their citizens and making better, future-proofed decisions and investments as communities and technologies evolve. The past two years confirmed that complexity is the ‘new normal’, we believe that the new normal model for smart cities to navigate is neural.
In practice
In practice, following the pandemic, we believe that city planners and managers need to reassess their smart city priorities and investments using the Neural Model as a map. This can be done in three ways:
- Reviewing smart city projects under development to challenge their alignment and investment cases against the Neural Model principles: will the use case and edge technology business cases really deliver the outcomes required when looked at in a new way?
- Mapping the city’s DNA, reviewing strategies for prioritising the dimensions and pillars and assessing the city’s current foundational technology status and investment roadmaps: are the fundamentals well enough understood?
- Embedding the principles of the Neural Model into smart city planning policies and procedures, and aligning stakeholders around these.
Image: Jamesteohart | Dreamstime.com