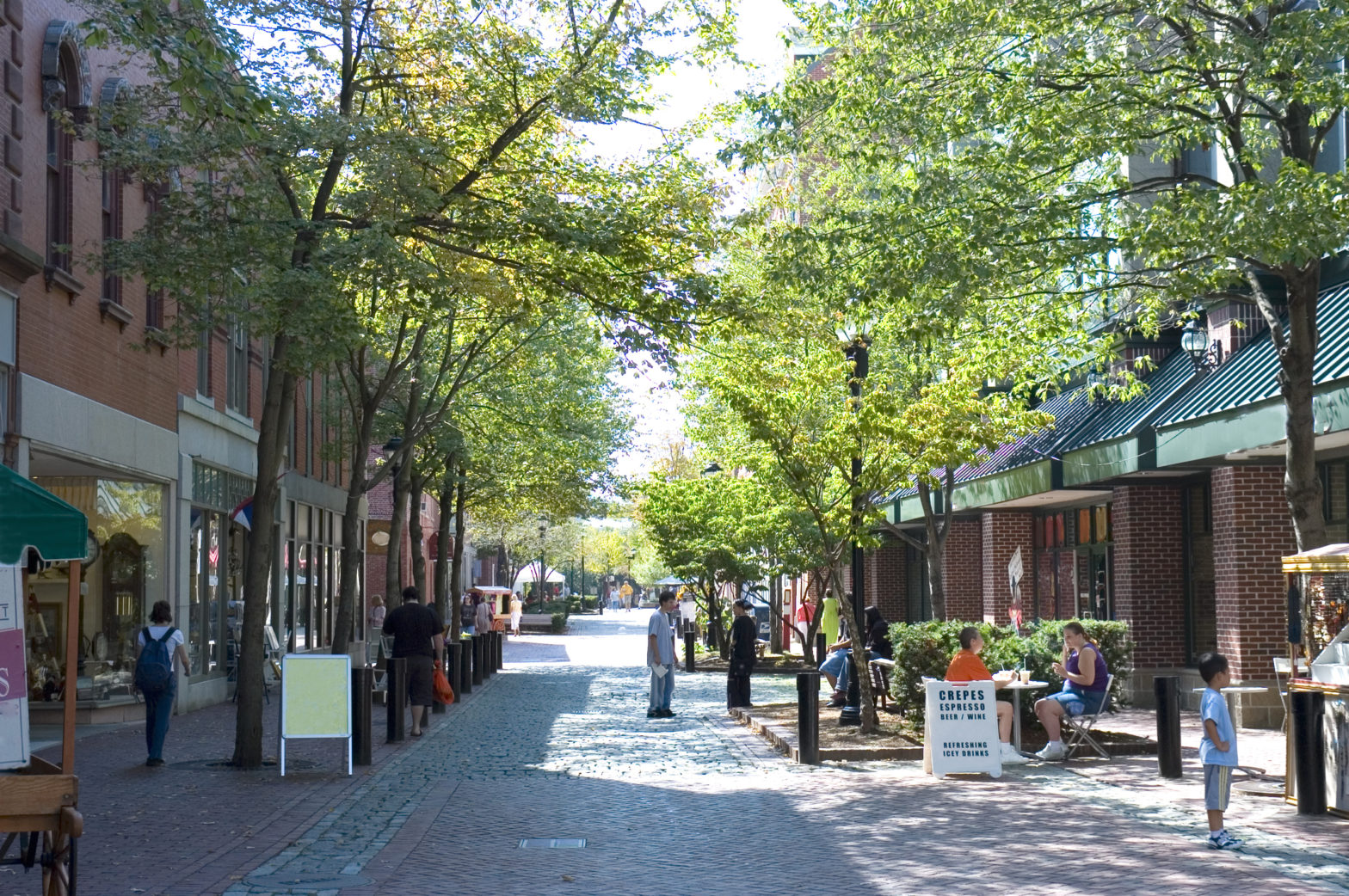
How AI could improve city planning
04 November 2024
by Jonathan Andrews
New research shows that using generative artificial intelligence such as ChatGPT and Google’s Gemini could improve city planning by enhancing access to tools that help measure walkability, safety, lighting, and more.
Unlike traditional methods that require technical expertise or manual work, researchers from Virginia Tech’s College of Natural Resources and Environment found large language models (LLMs) offer a more accessible tool for users, making it easier for policy and planning stakeholders to use these models in small- to medium-sized cities for managing smart urban infrastructure.
“LLMs have a reliably high performance in auditing a set of built environment features,” Kee Moon Jang, lead author of the study, told Cities Today. “The development of a user-friendly tool can significantly contribute to the productivity of local governments by reducing the time and cost involved in built environment audits.”
One example the researchers explored was to evaluate how walkable or bikeable a particular area is. The team had AI detect built environment features—benches, sidewalks, trees, and streetlights – all elements that influence how people perceive walkability and safety.
Trees, bicycles, roads and vegetation were features that both LLMs accurately identified (more than 95 percent), whereas streetlights, sidewalks and vehicles were the three least agreed among the 10 urban features included in the study (between 70 to 85 percent success rates).
Other limitations include biases in the AI’s training data, which can cause geographic disparities. The tools tend to perform better in large cities than in smaller towns because of the uneven availability of data to train the AI models.
“A significant takeaway is the potential need to shift focus toward assuring data quality,” added Jang. “Although street-view imagery has been highlighted as a reliable source for built environment audits, its limited spatial coverage and high temporal variability were found unsatisfactory. The better the data quality, the better the LLMs perform, which has encouraged researchers to create high-quality synthetic training data to improve these models.”
The study offers local geographers and urban planners a critical policy tool to explore underrepresented communities and can help identify areas that require intervention to promote community health and neighbourhood walkability despite the user’s level of technical proficiency.
Jang warns, however, that he is well aware of the geographical biases in the information used to train LLMs and calls for attentive use of such LLM-based methods to avoid disproportionate representation and application in practice.
The research was published in early October 2024 in The Professional Geographer and was done in collaboration with Kee Moon Jang with the Massachusetts Institute of Technology.
Image: Pierrette Guertin | Dreamstime.com