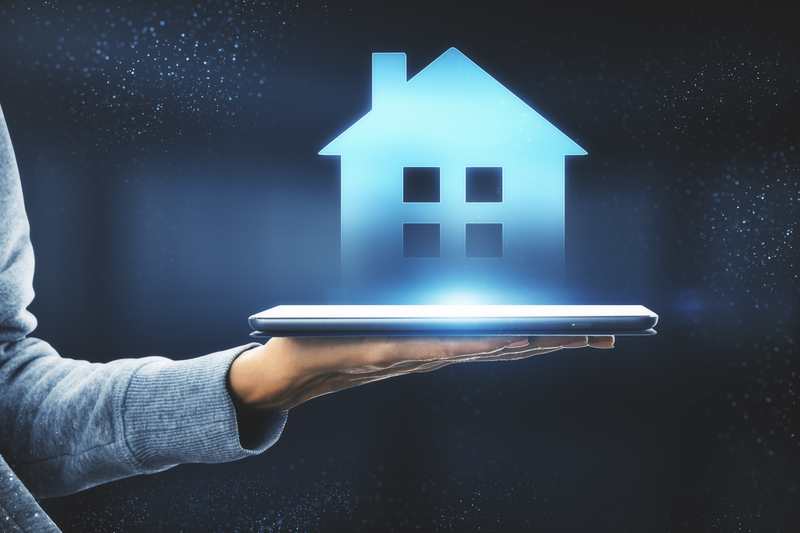
Photo: Daniil Peshkov | Dreamstime.com
‘Explainable AI’ predicts homelessness in Ontario city
25 August 2020
by Sarah Wray
The City of London in Canada is implementing an artificial intelligence (AI) tool it has developed internally to predict and prevent homelessness.
The Chronic Homelessness Artificial Intelligence (CHAI) model uses machine learning to forecast the probability of an individual in the city’s shelter system becoming chronically homeless within the next six months – that is, remaining in the shelter system for more than 180 days in a year. In July, 312 people in London were chronically homeless.
The tool was developed in-house with support from a consultant, and could help other cities – particularly those in Canada – deploy similar systems quickly.
The CHAI model grew out of London’s adoption of the federal Homeless Individuals and Families Information System (HIFIS), which is designed to provide a clearer picture of homelessness in communities and support organisations to work collaboratively. Using the software, London implemented a single database shared by 24 programmes involved in addressing homelessness, including shelters, housing initiatives and outreach teams.
Shared front
Jonathan Rivard, Manager, Homeless Prevention, City of London, told Cities Today: “[HIFIS] was the lever for us to move from siloed community support towards an integrated system and a shared front against homelessness. That was the springboard for the CHAI model.”
During the testing phase, the CHAI model, which uses information from the database, was able to predict chronic homelessness with 93 percent accuracy. The system is also helping to provide more local context about issues which contribute to homelessness, Rivard said. For example, factors identified in London include being male, over the age of 52, single, childless and without a source of income. People who haven’t self-resolved their homelessness within 30 days are also at a higher risk.
“Those are the individuals that we would like to know about as early on as possible,” Rivard commented. “If we are able to know within a week or two of their stay at a shelter that a person is very likely to stay in that shelter for six months a year or more, that helps us prioritise services and resources in supporting [them] to find housing.”
AI and decision-making
‘Explainable AI’ is an important aspect of the CHAI system, says Matt Ross, Manager, Artificial Intelligence, Information Technology Services, City of London. He believes the approach offers a “path forward” for the use of AI in government.
“A big barrier to implementing machine learning or artificial intelligence – particularly in public services – is the concept of a ‘black box’ model, where you give it data and it gives you a prediction but you don’t know why,” Ross said. “It’s hard to trust that, especially when you’re accountable as a public servant.”
Ross and his team designed the model around the principles of the General Data Protection Regulation (GDPR), though the legislation is not in force in Canada, as well as the federal government’s Directive on Automated Decision-Making.
“We built something that allows the model to explain what features it was relying on when it made a prediction,” said Ross. “This will be key if you’re prioritising resources and you want to do an audit of why a model made a prediction.”
He said that an “iterative” building process enables the team to understand and eliminate any unintended bias. The CHAI model is also “consent-driven” and allows people to opt out of their data being included.
Rivard points out, too, that: “If this was a tool that made decisions for us, I think there would be a need for a much deeper conversation around ethics, but this is just a tool to support decision-making.”
Integration
There are several areas where the CHAI model could now be applied in London to help reduce homelessness. An early application will be in the coordinated access system, which acts as an initial point of contact for citizens experiencing homelessness and directs them to appropriate services. Further down the line, the tool could be deployed in shelter services too.
“We’re still exploring the best avenues for using [it],” Rivard said. “There are some areas [where it would be] more easily implemented than others, and some that will require much more conversation, training and preparation to ready the community to incorporate the tool.”
There are other initiatives – including in Montreal in Canada, and Austin, New York and Los Angeles in the US – which use AI to predict homelessness but Ross said these are mainly at the research or prototyping phase, whereas London’s is now being integrated into service delivery.
He said this integration is key to success, including putting in place plans and processes for project management, maintenance and regular AI retraining. Data quality is also fundamental.
“It’s important to understand that you can’t just deploy a model and expect it to work well if you don’t have good data governance and data cleanliness principles. That heavy lifting is critical,” Ross commented.
Rivard said a lot of work had been done over recent years to create standards of practice with shelters and outreach teams to ensure data is being entered and used consistently.
The CHAI model has been developed in a modular way, along with detailed documentation. Once it has been live for some time and further assessed, the code will be made available for other municipalities to re-use. It could be particularly useful for Canadian cities which also use HIFIS.
Image: Daniil Peshkov | Dreamstime.com