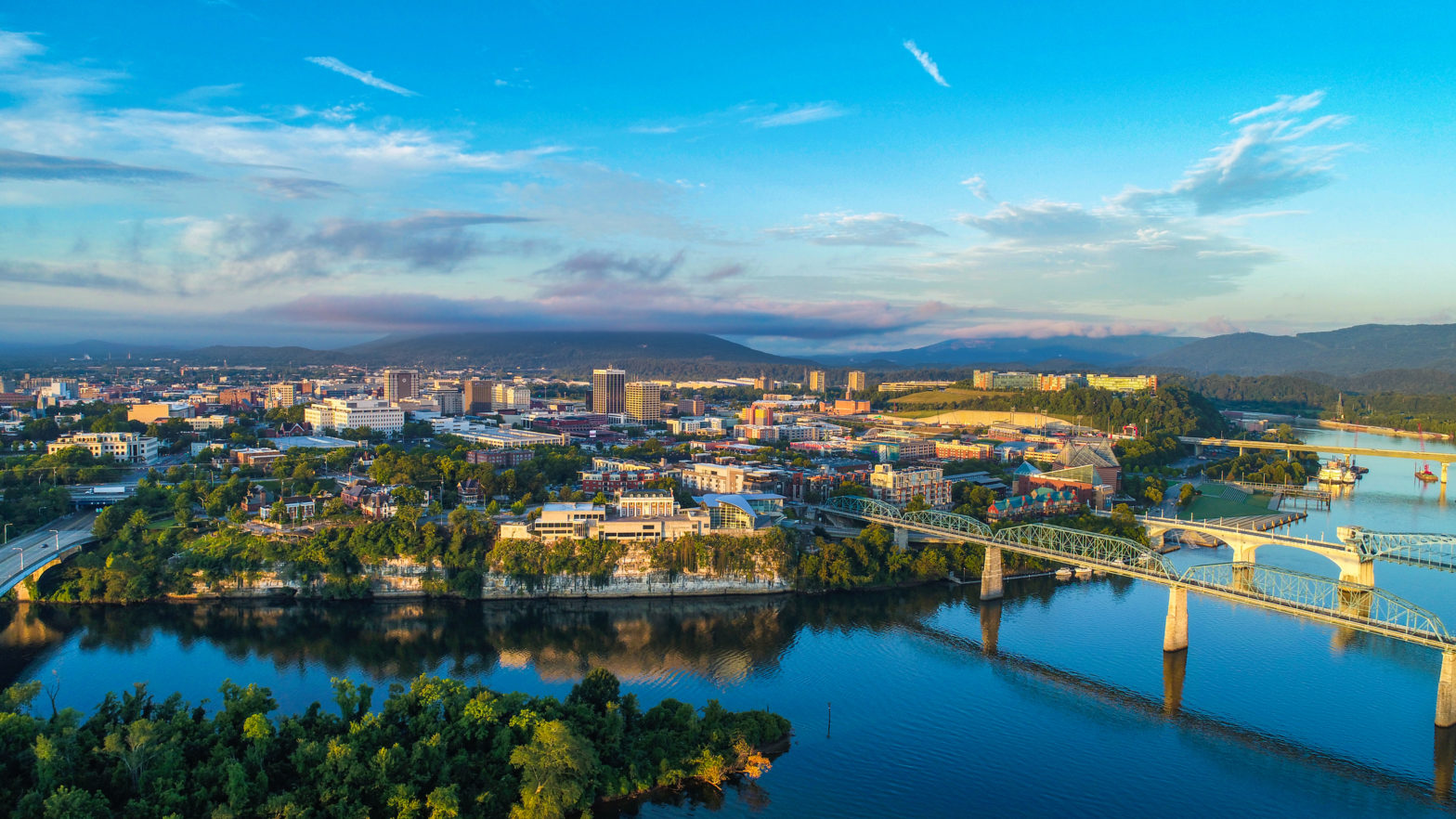
Cornell tests AI in Chattanooga mobility trial
04 April 2025
by William Thorpe
Chattanooga is working with Cornell University and other partners on a new initiative to test AI-driven neighbourhood mobility systems designed to improve the efficiency and uptake of public transport.
Funded by a US$3.2 million grant from the US Department of Energy, the project will introduce multimodal transport hubs in selected neighbourhoods. These ‘mobility zones’ in the Tennessee city will integrate buses, on-demand shuttles, electric vehicles and bike shares, coordinated through AI to recommend optimal routes and modes of transport to users in real time.
Cornell researchers are contributing to both the demand and supply sides of the project. On the demand side, Professor Ricardo Daziano, from Cornell’s Civil and Environmental Engineering Department, is leading the development of a choice-based recommender system that applies economic modelling to align transport options with individual preferences and constraints.
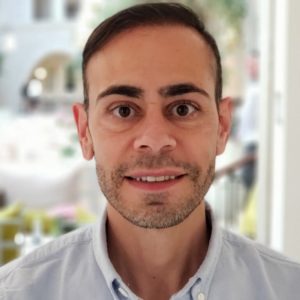
“Our system will exploit economic modelling to align individual preferences with real-time supply, allowing agencies to optimise service based on actual behavioural patterns–not just forecasts,” Daziano told Cities Today. “We expect the choice-based recommender system will provide a scalable tool to make public transport more responsive, efficient, and centred on user experience.”
With public transport accounting for just 1.6 percent of trips in Chattanooga, the project includes a focus on behavioural incentives to encourage mode shift.
“Emerging research is finding that personalised incentives–like targeted discounts and bundled subscriptions–can meaningfully shift travel behaviour,” he explained. “When shared mobility becomes more convenient and rewarding, people should be more willing to leave their cars behind. However, car use is deeply entrenched, and shifting away from it requires sustained engagement and a truly competitive alternative.”
Daziano noted that introducing AI into existing systems need not be disruptive.
“Start small and build incrementally,” he added. “Pilot programmes in defined zones, open data standards, and modular system integration are practical ways to bring AI into existing transport networks without disruption.”
The model is intended to be scalable and transferable, though implementation will vary depending on local conditions.
“Scalability depends on how well the model adapts to local conditions,” he said. “Factors like existing transport infrastructure, land use, and user behaviour will shape implementation.”
As cities continue to evaluate how best to integrate AI with public mobility systems, Daziano emphasised the importance of flexibility and data-driven planning.
“But the core idea–using economic modelling to personalise and optimise mobility–can be transferred, as long as cities invest in good data and maintain flexibility in service design.”
Image: Kevin Ruck | Dreamstime.com