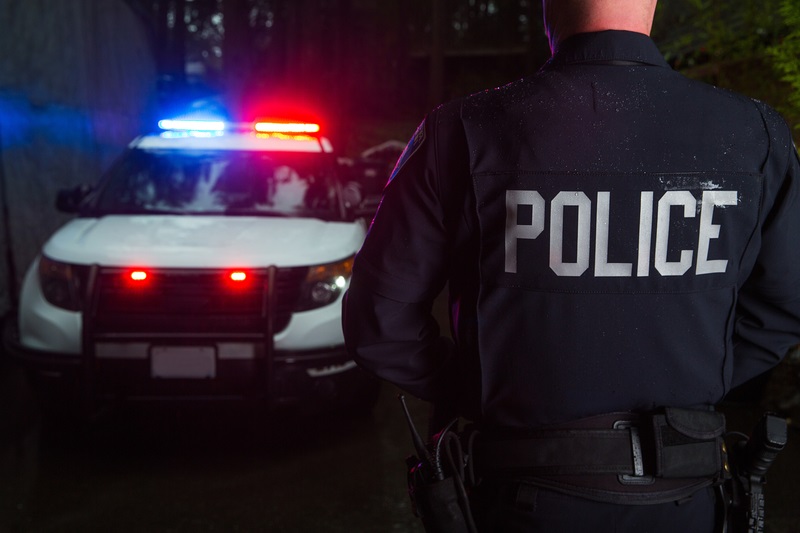
Photo: Mathayward | Dreamstime.com
Algorithm reignites debate on crime prediction
07 July 2022
by Sarah Wray
Researchers at the University of Chicago have developed an algorithm that they say can forecast the location and rate of crime across a city a week in advance.
The data and social scientists acknowledge that crime prediction tools have previously “stirred controversy” about bias and surveillance and say they have taken several steps to mitigate these risks. However, other experts still have concerns about how such tools could be used.
The model divides the city into spatial tiles roughly 1,000 feet across and ‘predicts’ crime within these areas. It was tested and validated using historical data from the City of Chicago around violent and property crime. According to the study published in Nature Human Behavior, the model can predict future crimes one week in advance with around 90 percent accuracy and performed just as well with data from seven other US cities: Atlanta, Austin, Detroit, Los Angeles, Philadelphia, Portland, and San Francisco.
It can predict, for example, within a two-block radius where there is a high risk of a homicide in a week’s time, Ishanu Chattopadhyay, Assistant Professor of Medicine at UChicago and senior author of the study, told Cities Today.
Real-life sci-fi?
Chattopadhyay said the tool shouldn’t be compared to Minority Report, a science fiction short story and film set in the future where people are arrested for crimes that haven’t been committed yet.
“We do not focus on predicting individual behaviour,” he said.
Previous crime prediction initiatives have been controversial, such as a now-abandoned programme in Chicago that rated tens of thousands of residents on who was most likely to be caught up in violence, either as a victim or criminal.
The team stresses that their approach differs from other efforts which depict crime as emerging in “hotspots” that spread to surrounding areas. They argue that these tools miss out on the complex social environment of cities, and don’t consider the relationship between crime and the effects of police enforcement.
The tool can also be used to audit bias. The researchers studied the police response to crime by analysing the number of arrests following incidents and comparing those rates among neighbourhoods with different socioeconomic status. They saw that crime in wealthier areas resulted in more arrests than in poorer neighbourhoods.
According to Chattopadhyay, the open nature of the model further sets it apart. “The data is public, the algorithm is open source, and consequently the results may be replicated by anyone with access to a moderately powerful computing setup,” he says.
Machine learning algorithms often need “features” as inputs, which are determined by data science or domain experts. The new algorithm has no requirement for this human input as it processes the raw event logs directly.
“This might be seen as a step towards democratisation of AI: there are no hidden inputs, no data annotations that are privy to the ‘authorities’, and no one is sitting down and keying in parameters that would then be used to define and identify bad or ‘at risk’ behaviours,” said Chattopadhyay.
Risks remain
“That does not mean we have eliminated potential bias,” he added. “The data might have bias built in. For example, over-policing disadvantaged communities will falsely elevate the relative crime rate, and the inference algorithm will not be able to tell. So we have to be careful how this, and such similar tools, are used.
“We must leverage this technology to help communities, to recognise biases where they exist, and move towards a better society, not flood disadvantaged communities with more enforcement.”
However, others point out that the creators of algorithms have little control over how they’re used in practice.
Vincent M. Southerland, an Assistant Professor of Clinical Law and Co-Faculty Director of the Center on Race, Inequality and the Law at NYU School of Law, told Cities Today: “I don’t envision police departments using this tool to figure out ways to allocate resources that actually address the underlying problems which lead to people’s involvement in the criminal legal system.
“I certainly see it being used to figure out where to deploy police resources. And in that vein, I think it’s going to contribute to the same type of over-policing as we’ve seen in communities of colour, historically across the board.”
He said such tools can “cast a net of suspicion” over everyone in an area as a potential criminal.
Emily M. Bender, Professor of Linguistics at the University of Washington, posted a thread on Twitter saying that the focus should be on targeting underlying inequities rather than prediction and noting that crimes such as wage theft, financial fraud and environmental violations typically receive less attention.
Chattopadhyay said: “We are being cautious on deployment given the many, many unintended consequences that such technology might have. But the tool itself is pretty complete and can be deployed quickly.
“We are putting together the best avenue for that, but we might see some deployments of this tool within a year.”